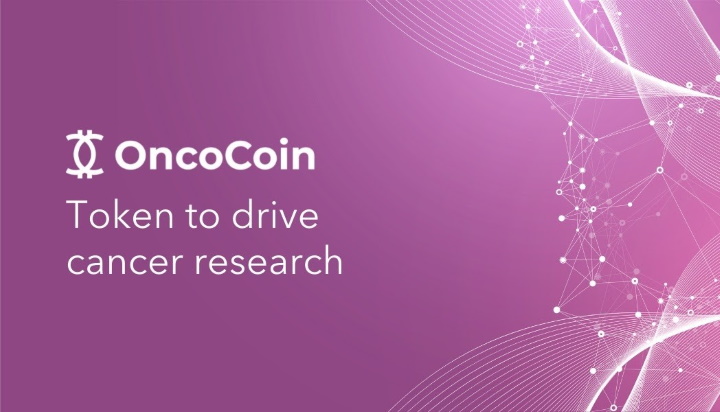
Artificial Intelligence (AI) is one of the most diverse, agile and promising emerging technologies, and is said to be the crux of the ongoing fourth industrial revolution. When we think of AI and automation, our minds wander to use cases like robotics, self-driving cars, autonomous supply chains, and so on. However, one of the most significant applications of AI and automation is in healthcare.
Data is the key to unlocking the true potential for AI, machine learning, and deep learning; but data collection in the healthcare sector has always been a hurdle in accelerating the use of AI in the domain. Data is often outdated, biased or incomplete, and this lack of adequate data has hindered the progression of drug discovery, specifically in the field of cancer.
AI in cancer research
Cancer research is one of the most popular fields in the healthcare research sector. AI is already being used to accelerate cancer research. Infact, AI is used to uncover insights from previous medical research to form the basis of new hypotheses. Furthermore, AI is being used to map and personalize a patient's cancer journey, helping to identify the correct treatment options. AI is even being trained to predict an individual's chances of getting a particular type of cancer.
One example of AI and machine learning application in cancer research is Ontosight, offering a comprehensive approach to research and discovery in life sciences using AI and its self-learning ontology. Researchers get real-time, relevant insights from modules and dashboards that help to accelerate processes throughout the clinical research lifecycle, and thereby reduce resource inefficiencies during the research, bringing therapies to patients faster.
AI in cancer treatment
Currently, some of the most promising work on AI in oncology is taking place within the area of cancer imaging, specifically digital pathology, radiographic imaging, and clinical photographs. In digital pathology, AI has been applied to both low and high-level image processing and classification tasks to automate time-consuming tasks and to improve diagnostic accuracy.
AI is also being used to help improve diagnostic accuracy and reduce diagnostic uncertainty among dermatologic and gastrointestinal malignancies on clinical imaging. AI can provide accurate estimates of a patient's risk for experiencing a host of outcomes, including rehospitalization, cancer recurrence, treatment response, pharmacogenomics, and patient Overall Survival Rates. Predictive analytics may aid the delivery of oncology treatments to populations that are disadvantaged or underrepresented in clinical trials for whom it is challenging to apply for evidence-based medicine.
Another application of AI involves precision oncology. The rapid growth of genomic tumor characterization has led to the development of computational methods to aid the interpretation of these data and to aid precision oncology.
Although there has been some progress made with AI in cancer research and treatment, gathering adequate, structured and diverse data have been bottlenecks in this process.
AI in cancer research has its limitations currently
Even though AI in cancer research has evolved across multiple paradigms, there are significant barriers which remain to be overcome. Healthcare data is often recorded and stored in highly heterogeneous, multimodal, and unstructured ways leading to problems in data standardization collection and management.
Additionally, AI in its current form is essentially focused on recognizing patterns. However, healthcare data formats are diverse and complex therefore it’s difficult to find common patterns or build models which can be reused over a new type of data. This means the AI models on such healthcare data cannot be easily generalized and scaled.
On top of this, the absence of explainability in AI models has contributed to a trust deficit, which could limit the widespread adoption of AI. AI algorithms, especially deep learning algorithms, are sensitive to subtle nuances in the data that cannot be identified, meaning a lack of reproducibility is a real possibility that could be hard to overcome.
OncoCoin is an ecosystem focussing on accelerating drug discovery by optimising the way research data is collected and analysed, through a democratised approach.
OncoCoin collects high-quality patient data for cancer research
The OncoCoin ecosystem aims to bring the power of data crowdsourcing to the world of oncology and clinical research in general.
The ecosystem empowers the patients by giving them an overview of treatment options, clinical trials, and the best available experts a precious second opinion, all tailored to their cancer type and stage. This information is extracted from billions of webpages daily using artificial intelligence, to ensure patients have an unbiased, accurate and up-to-date source of cancer information at their fingertips.
Symbiotically, the ecosystem helps research projects get access to much-needed Real-World Data (RWD) that is standardized to be further analyzed and broken down easily.
RWD is key as an additional source to secondary data, to help train AI. Proprietary RWD gives the AI more sources to learn from, expanding the ontology of the tool. With this RWD, AI will be able to more accurately predict novel and life-saving therapies.
Through OncoCoin, researchers can submit projects where they have data gaps and RWD from the patients in the OncoCoin ecosystem can help advance their research. Patients can choose the projects where their data can be licensed while being in total control of it, and in return they can be incentivized with the OncoCoin utility token for doing so.
The data licensed by patients in the OncoCoin ecosystem is analogous and equivalent, so that each patient's data can be compared to the others. This homogeneity helps in OncoCoin's proprietary AI tool which in turn accelerates the drug discovery process for patients.
OncoCoin, through crowdsourcing of Real World Data, incentivizes patients in the process. It is working to solve the much-needed problem of curated data generation plaguing the healthcare industry in large; the sort of data that seemed to be a hurdle in the application of AI and machine learning. Cancer research and drug discovery can break through new horizons while creating a win-win scenario for everyone involved in the ecosystem.